A wafer full of memristors. Image Credit: Courtesy of UCL
Extremely energy efficient artificial intelligence is now closer to reality after a study by UCL researchers found a way to improve that accuracy a brain-inspired computer system.
The system that uses memristors to create artificial neural networks is at least 1000 times more energy efficient than conventional transistor-based AI hardware, but has so far been more error-prone.
Existing AI is extremely energy-intensive – training an AI model can generate 284 tons of carbon dioxide, which is equivalent to the lifetime emissions of five cars. By replacing the transistors that make up all digital devices with memristors, a novel electronic device first built in 2008, this could be reduced to a fraction of a ton of carbon dioxide – the equivalent of the emissions produced by an afternoon drive .
Because memristors are so much more energy efficient than existing computer systems, they can potentially pack large amounts of computing power into handheld devices so that they do not need to be connected to the Internet.
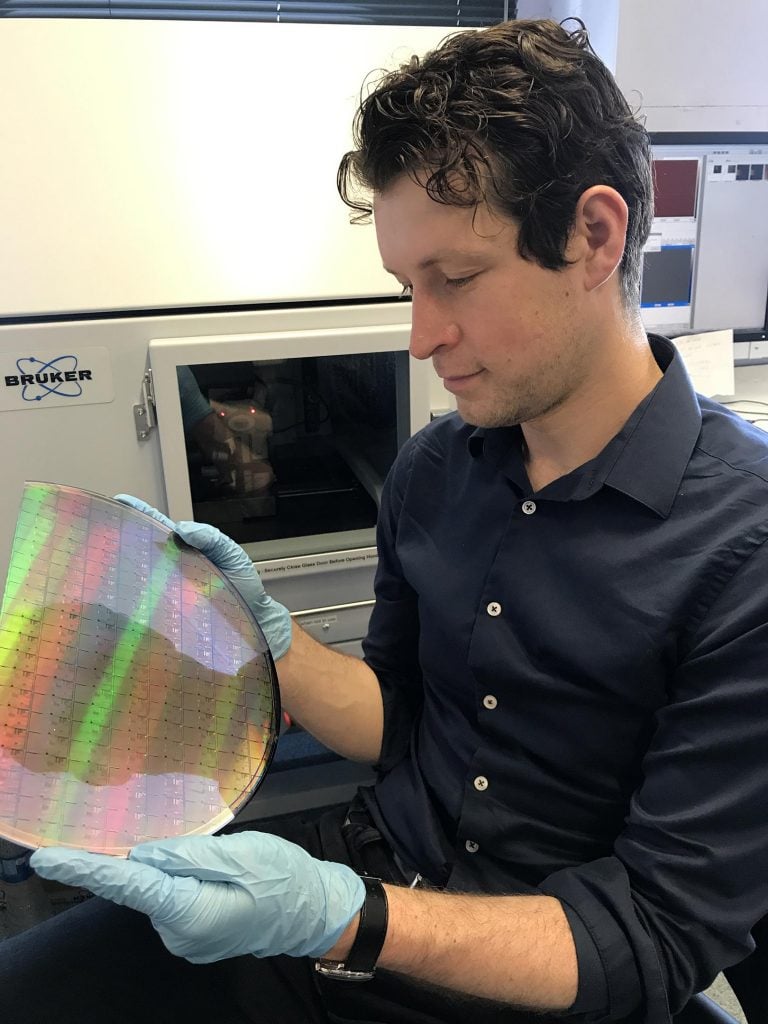
Dr. Adnan Mehonic holds a wafer with memristors in his hand. Image Credit: Courtesy of UCL
This is especially important as over-reliance on the Internet is likely to become problematic in the future due to the ever increasing data requirements and the difficulty of increasing data transfer capacity beyond a certain point.
In the new study published in Communication with natureUCL engineers found that accuracy could be improved significantly by having memristors in multiple subgroups of neural networks work together and averaged their calculations, meaning that errors in each of the networks can be fixed.
Memristors, known as “memory resistors” because they remember the amount of electrical charge that passed through them after they were turned off, were considered revolutionary when they were first built over a decade ago “Missing link” in electronics as a supplement to the resistor, capacitor and inductor. They have since been made commercially in storage devices, but the research team says they could be used to develop AI systems within the next three years.
Memristors offer vastly improved efficiency, as they not only work in a binary code of ones and zeros, but at multiple levels between zero and one at the same time, allowing more information to be packed into each bit.
In addition, memristors are often described as a neuromorphic (brain-inspired) form of computing, as processing and storage are implemented in the same adaptive building blocks as in the brain, unlike current computer systems which waste a lot of energy on data.
In the study, Dr. Adnan Mehonic, PhD student Dovydas Joksas (both UCL Electronic & Electrical Engineering) and colleagues from the UK and US took the new approach to different types of memristors and found that it improved the accuracy of all them, regardless of material or particular memristor technology. It has also worked on a number of different problems that can affect the accuracy of the memristors.
The researchers found that their approach increased the accuracy of neural networks for typical AI tasks to a level comparable to that of software tools that run on traditional digital hardware.
Dr. Mehonic, director of the study, said, “We were hoping there could be more general approaches that improve behavior at the system level rather than at the device level, and we believe we have found one. Our approach shows that when it comes to memristors, several heads are better than one. Arranging the neural network in several smaller networks instead of one large network resulted in an overall higher accuracy. ”
Dovydas Joksas continued: “We borrowed a popular technique from computer science and applied it in the context of memristors. And it worked! Using preliminary simulations, we found that even a simple averaging can significantly improve the accuracy of memristive neural networks. ”
Professor Tony Kenyon (UCL Electronic & Electrical Engineering), co-author of the study, added, “We believe the time is now for memristors, which we’ve been working on for several years, to lead the way in making more energy – sustainable Era of IoT devices and edge computing. ”
Reference: “Committee machines – a universal method for dealing with non-idealities in memristor-based neural networks” by D. Joksas, P. Freitas, Z. Chai, WH Ng, M. Buckwell, C. Li, WD Zhang, Q. Xia , AJ Kenyon and A. Mehonic, August 26, 2020, Nature communication.
DOI: 10.1038 / s41467-020-18098-0